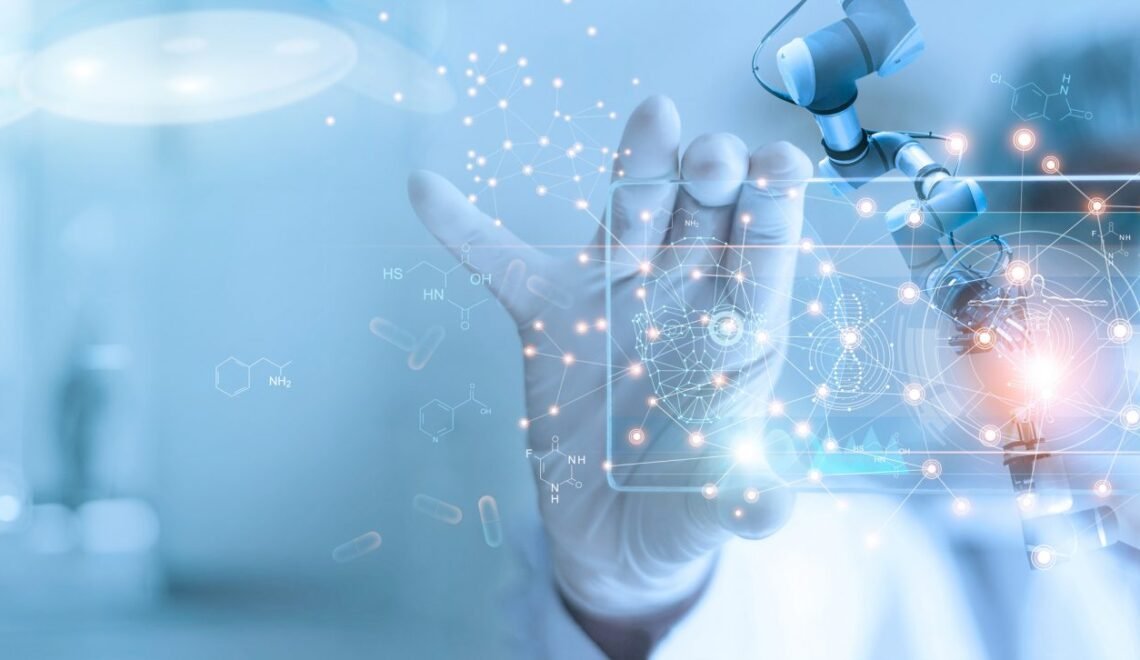
For countless years, in-vitro fertilisation (IVF) has presented individuals who are unable to conceive naturally with the opportunity to have children of their own. Nonetheless, the attainment of satisfactory results on a global scale has been famously limited. The employment of artificial intelligence (AI) is being pursued as a means to enhance the success rates of IVF, although it is not free from obstacles. Can AI potentially serve as a valuable tool within clinical settings to augment the effectiveness of IVF?
In recent decades, technological advancements have significantly influenced various aspects of healthcare, and one notable area is assisted reproductive technology (ART). Among these technologies, In Vitro Fertilization (IVF) has revolutionized the landscape of fertility treatment. As science and medicine continue to evolve, the integration of Artificial Intelligence (AI) into IVF procedures has emerged as a promising avenue to enhance success rates, streamline processes, and ultimately improve outcomes for couples struggling with infertility.
(I. Understanding In Vitro Fertilization
A. Background of IVF
1. Historical development
2. Prevalence and growth of IVF procedures globally
B. IVF Procedure Overview
1. Stages of IVF
2. Key challenges and limitations) >>>> All explained in earlier blogs
II. The Current Landscape of Artificial Intelligence
A. Overview of Artificial Intelligence
1. Definition and key concepts
Artificial Intelligence (AI) refers to the simulation of human intelligence in machines that are programmed to think and learn like humans. It involves the development of algorithms and models that enable computers to perform tasks that typically require human intelligence. Key concepts in AI include:
1. Machine Learning (ML): A subset of AI that focuses on the development of algorithms and statistical models that enable computers to improve their performance on a task through experience. It includes supervised learning, unsupervised learning, and reinforcement learning.
2. Deep Learning (DL): A subfield of machine learning that involves the use of artificial neural networks to model and solve complex problems. Deep learning has been particularly successful in tasks such as image and speech recognition.
3. Natural Language Processing (NLP): The ability of machines to understand, interpret, and generate human language. NLP is crucial for applications like chatbots, language translation, and sentiment analysis.
4. Computer Vision: The field of AI that focuses on enabling machines to interpret and understand visual information from the world, such as images and videos. It is widely used in image recognition, object detection, and video analysis.
5. Robotics: The integration of AI technologies into physical systems, allowing machines to perceive their environment, make decisions, and take actions. Robotics is applied in various domains, including manufacturing, healthcare, and autonomous vehicles.
6. Expert Systems: AI systems that emulate the decision-making abilities of a human expert in a specific domain. These systems use a knowledge base and a set of rules to draw inferences and make decisions.
7. Reinforcement Learning: A type of machine learning where an agent learns to make decisions by interacting with an environment. The agent receives feedback in the form of rewards or penalties based on its actions, and it adjusts its behavior to maximize cumulative reward.
8. Data Mining: The process of discovering patterns and knowledge from large datasets. Data mining techniques are often used in AI to extract valuable insights and make predictions.
9. Algorithmic Bias: The presence of biases in AI models that can lead to unfair or discriminatory outcomes. Addressing algorithmic bias is a crucial aspect of responsible AI development.
10. Explainable AI (XAI): The effort to make AI systems more transparent and understandable by providing explanations for their decisions. This is important for building trust and ensuring accountability in AI applications.
11. Ethical AI: The consideration of ethical principles and values in the development and deployment of AI systems. This includes issues related to privacy, fairness, accountability, and the societal impact of AI.
Understanding these key concepts is essential for anyone working with or studying artificial intelligence, as they form the foundation for the various applications and advancements in this rapidly evolving field.
2. Applications in various industries
AI (Artificial Intelligence) has found applications across various industries, transforming processes, improving efficiency, and enabling new capabilities. Here are some examples of AI applications in different sectors:
1. Healthcare:
– Diagnosis and Imaging: AI assists in medical image analysis for better diagnostics.
– Drug Discovery: Accelerates the drug discovery process by analyzing complex biological data.
– Personalized Medicine: Tailors treatment plans based on individual patient data.
2. Finance:
– Fraud Detection: Identifies unusual patterns and anomalies in financial transactions.
– Algorithmic Trading: Uses AI algorithms for automated trading and investment strategies.
– Credit Scoring: Analyzes customer data for more accurate credit risk assessment.
3. Retail:
– Recommendation Systems: Enhances customer experience through personalized product recommendations.
– Inventory Management: Optimizes inventory levels with predictive analytics.
– Supply Chain Optimization: Improves logistics and supply chain efficiency through predictive analytics.
4. Manufacturing:
– Predictive Maintenance: Anticipates equipment failures to reduce downtime.
– Quality Control: Uses computer vision and machine learning for automated quality inspections.
– Process Optimization: Optimizes production processes for efficiency and cost reduction.
5. Automotive:
– Autonomous Vehicles: Enables self-driving cars through advanced AI algorithms.
– Predictive Maintenance: Anticipates vehicle maintenance needs based on usage data.
– Traffic Management: Optimizes traffic flow and reduces congestion in smart cities.
6. Education:
– Personalized Learning: Adapts educational content based on individual student performance.
– Automated Grading: Streamlines the grading process for assignments and exams.
– Student Support: Provides virtual assistants and chatbots for student queries.
7. Telecommunications:
– Network Optimization: Improves network performance and efficiency.
– Customer Service Chatbots: Enhances customer support with AI-powered virtual assistants.
– Predictive Analytics: Analyzes data to anticipate network issues and optimize resource allocation.
8. Energy:
– Predictive Maintenance: Enhances the reliability of energy infrastructure.
– Grid Management: Optimizes energy distribution and consumption in smart grids.
– Energy Forecasting: Predicts energy demand for efficient resource planning.
9. Agriculture:
– Precision Farming: Utilizes AI and IoT for optimized crop management.
– Crop Monitoring: Analyzes satellite and drone imagery for crop health assessment.
– Predictive Analytics: Forecasts crop yields and identifies potential issues.
10. Entertainment:
– Content Recommendation: Recommends movies, music, and other content based on user preferences.
– Virtual Production: Enhances special effects and animation in the film and gaming industries.
– AI-generated Content: Creates AI-generated music, art, and other creative works.
These examples showcase the diverse applications of AI across industries, and as technology continues to advance, new and innovative uses are likely to emerge.
B. AI in Healthcare
1. Diagnostic tools and medical imaging
AI (Artificial Intelligence) has made significant strides in the field of diagnostic tools and medical imaging, offering innovative solutions to enhance accuracy, efficiency, and overall healthcare outcomes. Here are some key areas where AI is being applied in diagnostic tools and medical imaging:
1. Image Recognition and Analysis:
– Computer-Aided Diagnosis (CAD): AI algorithms analyze medical images, such as X-rays, CT scans, and MRIs, to assist radiologists in detecting abnormalities or potential diseases.
– Pathology Imaging: AI helps pathologists analyze tissue samples by identifying patterns and anomalies in microscopic images, aiding in the diagnosis of diseases like cancer.
2. Radiology:
– Image Interpretation: AI algorithms can interpret radiological images, assisting radiologists in detecting and diagnosing conditions earlier and with greater accuracy.
– Predictive Analytics: AI can predict disease progression and outcomes based on historical imaging data, aiding in treatment planning and decision-making.
3. Dermatology:
– Skin Lesion Classification: AI systems can analyze dermatological images to identify and classify skin lesions, assisting dermatologists in early detection of skin cancers.
4. Cardiology:
– Echocardiography and Cardiac Imaging: AI is used for analyzing cardiac images, helping to detect heart diseases, assess cardiac function, and identify abnormalities.
5. Neurology:
– Brain Imaging: AI assists in the analysis of brain scans, such as CT and MRI, for early detection of neurological disorders, including tumors and degenerative diseases.
– Stroke Detection: AI algorithms can quickly analyze imaging data to identify signs of stroke, enabling faster intervention and treatment.
6. Ophthalmology:
– Retinal Imaging: AI is employed for the analysis of retinal images to detect conditions such as diabetic retinopathy and age-related macular degeneration.
7. Mammography:
– Breast Cancer Screening: AI algorithms can aid in the interpretation of mammograms, improving the accuracy of breast cancer detection.
8. Genomic Medicine:
– Genomic Data Analysis: AI helps analyze vast amounts of genomic data to identify genetic markers associated with specific diseases, facilitating personalized medicine approaches.
9. Chatbots and Symptom Checkers:
– AI-powered chatbots and symptom checkers assist in preliminary diagnosis by asking patients about their symptoms and medical history, providing initial guidance.
10. Drug Discovery:
– AI is used to analyze complex biological data, accelerating drug discovery and development processes.
11. Remote Monitoring:
– AI facilitates remote monitoring of patients through wearable devices, analyzing real-time data to detect abnormalities and provide timely interventions.
Despite these advancements, it’s essential to validate and ensure the safety and reliability of AI algorithms in healthcare settings. Ethical considerations, privacy concerns, and regulatory frameworks also play a crucial role in the responsible deployment of AI in diagnostic tools and medical imaging.
2. Personalized medicine and treatment plans
Personalized medicine, also known as precision medicine, involves tailoring medical treatment and healthcare decisions to individual characteristics of each patient. Artificial Intelligence (AI) plays a crucial role in advancing personalized medicine by analyzing large datasets, identifying patterns, and making predictions based on individual patient information. Here are some ways AI contributes to personalized medicine and treatment plans:
1. Genomic Analysis:
– AI algorithms can analyze a patient’s genomic data to identify genetic variations associated with specific diseases or drug responses.
– This information helps in designing targeted therapies based on the patient’s genetic makeup, increasing the effectiveness of treatment.
2. Disease Prediction and Risk Assessment:
– AI models can analyze patient data, including genetic information, lifestyle factors, and medical history, to predict the risk of developing certain diseases.
– Early identification of risks allows for proactive interventions and personalized prevention strategies.
3. Drug Discovery and Development:
– AI accelerates drug discovery by analyzing biological data and predicting potential drug candidates.
– Virtual screening and molecular modeling help identify drugs that are likely to be effective for specific patient populations, improving treatment outcomes.
4. Treatment Response Prediction:
– AI algorithms analyze patient data to predict how individuals are likely to respond to specific treatments.
– This information assists healthcare providers in selecting the most effective therapies and avoiding treatments that may be less beneficial or have adverse effects.
5. Tailored Treatment Plans:
– AI helps create personalized treatment plans by considering individual patient factors, such as genetics, lifestyle, and environmental influences.
– Treatment plans can be dynamically adjusted based on real-time data, ensuring continuous optimization for the best possible outcomes.
6. Remote Patient Monitoring:
– AI-powered devices and wearables enable continuous monitoring of patients in real-time.
– This data is used to personalize treatment plans, detect early signs of deterioration, and make timely adjustments to healthcare interventions.
7. Clinical Decision Support Systems:
– AI-based decision support systems assist healthcare professionals in making personalized and evidence-based decisions.
– Integrating AI into electronic health records helps streamline data analysis and provides insights at the point of care.
8. Natural Language Processing (NLP) in Healthcare Records:
– AI-driven NLP systems extract valuable information from unstructured clinical notes and records, enhancing the depth of patient profiles.
– This contributes to a more comprehensive understanding of the patient’s health status and facilitates personalized treatment recommendations.
In summary, AI empowers personalized medicine by leveraging data-driven insights to tailor healthcare interventions to the unique characteristics of each patient. This approach holds great promise for improving treatment efficacy, reducing adverse effects, and ultimately enhancing patient outcomes.
III. The Intersection of AI and IVF
A. AI Applications in Reproductive Medicine
1. Predictive analytics for fertility assessment
Predictive analytics for fertility assessment using AI involves utilizing artificial intelligence (AI) algorithms and machine learning (ML) techniques to analyze various factors and predict fertility outcomes. Here’s an overview of how predictive analytics can be applied to fertility assessment:
1. Data Collection:
– Gather comprehensive data: Collect a diverse range of data related to fertility, including demographic information, medical history, lifestyle factors, menstrual cycle data, hormonal levels, and any available genetic information.
2. Feature Selection:
– Identify relevant features: Determine which variables or features are most influential in predicting fertility outcomes. This may involve statistical analysis or machine learning techniques to identify key factors.
3. Data Preprocessing:
– Clean and preprocess data: Address missing values, outliers, and normalize data to ensure the quality and consistency of the dataset. This step is crucial for the accuracy of predictive models.
4. Model Selection:
– Choose appropriate models: Select machine learning models suitable for the fertility prediction task. Common algorithms include decision trees, support vector machines, neural networks, and ensemble methods.
5. Training the Model:
– Train the AI model: Use historical data to train the chosen model. The model learns patterns and relationships within the data to make predictions about future fertility outcomes.
6. Validation and Evaluation:
– Validate the model: Assess the model’s performance using separate datasets not used during training. Common evaluation metrics include accuracy, precision, recall, and F1 score.
7. Iterative Improvement:
– Refine the model: Fine-tune the model based on feedback and performance metrics. This may involve adjusting hyperparameters, trying different algorithms, or incorporating additional features.
8. Deployment:
– Implement the model: Once the model demonstrates reliable performance, deploy it for real-world fertility assessments. This can be integrated into healthcare systems, fertility clinics, or mobile applications.
9. Continuous Monitoring:
– Monitor and update: Regularly monitor the model’s performance in real-world scenarios. Update the model as needed to account for changes in data patterns or shifts in the population.
10. Ethical Considerations:
– Address ethical concerns: Ensure that the use of AI in fertility assessments complies with ethical standards and patient privacy. Transparency and explainability of AI predictions are essential for gaining trust from users and healthcare professionals.
11. User Interface:
– Develop a user-friendly interface: Create an intuitive interface for users, such as healthcare providers or individuals seeking fertility assessments, to interpret and act upon the predictions made by the AI model.
By integrating predictive analytics with AI in fertility assessment, there is potential for more personalized and accurate predictions, allowing individuals and healthcare professionals to make informed decisions regarding fertility-related matters.
2. Automated image analysis for embryo selection
Automated image analysis for embryo selection using AI has become a promising and innovative approach in the field of assisted reproductive technologies (ART). This technology is commonly known as “embryo scoring” or “embryo selection,” and it involves using artificial intelligence (AI) algorithms to evaluate and rank embryos based on various morphological features observed in microscopy images. Here’s an overview of how AI is utilized in this context:
1. Image Acquisition:
– High-quality images of embryos are captured using specialized microscopes and imaging systems.
– Images may include details such as the number of cells, symmetry, fragmentation, and other morphological characteristics.
2. Data Preprocessing:
– Raw images may undergo preprocessing steps, including normalization, noise reduction, and image enhancement, to ensure optimal input for the AI algorithm.
3. Feature Extraction:
– Relevant features are extracted from the preprocessed images. These features could include parameters like the size and shape of cells, the degree of symmetry, and the presence of anomalies or irregularities.
4. Training the AI Model:
– An AI model, often based on deep learning techniques like convolutional neural networks (CNNs), is trained using a dataset of labeled embryo images.
– The labeled dataset typically includes information about the developmental success of embryos, allowing the AI model to learn the correlation between image features and successful outcomes.
5. Validation and Fine-Tuning:
– The trained model is validated using separate datasets to ensure its generalization to new, unseen data.
– Fine-tuning may be performed to optimize the model’s performance, adjusting parameters based on the feedback from validation results.
6. Embryo Scoring:
– The trained AI model is applied to analyze new embryo images.
– The model assigns a score or probability indicating the likelihood of a successful implantation based on the learned features.
7. Clinical Decision Support:
– The AI-generated scores can be used by embryologists as a tool for making informed decisions about which embryos to select for transfer.
Benefits of using AI in embryo selection include:
– Standardization of embryo assessment.
– Objective and consistent analysis.
– Potential improvement in pregnancy success rates.
However, it’s crucial to note that while AI can assist in decision-making, it should not replace the expertise of embryologists. It is often used as a complementary tool to support clinical decision-making rather than a standalone solution. Additionally, regulatory and ethical considerations should be taken into account when implementing AI in clinical settings.
B. Improving Fertility Assessment with AI
1. Predicting optimal fertility windows
Predicting optimal fertility windows using AI involves leveraging data-driven approaches to analyze various factors that influence fertility. Here are some steps and considerations for building a predictive model:
1. Data Collection:
– Gather relevant data such as menstrual cycle information, basal body temperature, hormone levels, lifestyle factors (e.g., diet, exercise), and any other relevant health information.
– Consider using wearable devices or mobile apps that track menstrual cycles, ovulation, and other fertility-related data.
2. Feature Selection:
– Identify key features that may influence fertility, such as cycle length, menstrual irregularities, body temperature variations, hormonal levels, age, and lifestyle factors.
– Ensure data privacy and ethical considerations are taken into account when handling sensitive health information.
3. Data Preprocessing:
– Clean and preprocess the data to handle missing values, outliers, and inconsistencies.
– Normalize or scale numerical features and encode categorical variables if necessary.
4. Model Selection:
– Choose a suitable machine learning model for the task. Common models include decision trees, random forests, support vector machines, and neural networks.
– Consider using time-series analysis techniques if you have data that includes a temporal component.
5. Training the Model:
– Split the dataset into training and testing sets to evaluate the model’s performance.
– Train the model using the training set, adjusting hyperparameters as needed.
– Implement techniques like cross-validation to ensure the model generalizes well to new data.
6. Validation and Evaluation:
– Validate the model using the testing set to assess its performance.
– Use metrics such as accuracy, precision, recall, and F1 score to evaluate the model’s predictive capabilities.
– Consider obtaining feedback from healthcare professionals to validate the model’s predictions against clinical knowledge.
7. Iterative Improvement:
– Continuously refine the model based on feedback and additional data.
– Incorporate new features or adjust existing ones to enhance predictive accuracy.
8. User-Friendly Interface:
– Develop a user-friendly interface or integrate the model into existing fertility tracking apps.
– Ensure clear communication of predictions, providing users with actionable insights.
9. Ethical Considerations:
– Address ethical concerns related to fertility predictions, such as providing accurate information, ensuring user consent, and maintaining data privacy.
10. Clinical Collaboration:
– Collaborate with healthcare professionals to validate and refine the model, ensuring alignment with medical standards.
It’s important to note that predicting fertility windows is a complex task, and the accuracy of the predictions may vary among individuals. Additionally, consulting with healthcare professionals is crucial for interpreting and acting upon the model’s predictions.
2. Analyzing physiological markers for fertility prediction
Predicting fertility using physiological markers involves analyzing various biological indicators to assess a person’s reproductive health. Artificial intelligence (AI) can play a significant role in this process by leveraging machine learning algorithms to analyze and interpret complex datasets. Here are some physiological markers commonly considered for fertility prediction and how AI can be applied:
1. Hormone Levels:
– Markers: Follicle-stimulating hormone (FSH), luteinizing hormone (LH), estrogen, and progesterone.
– AI Application: AI algorithms can analyze patterns and fluctuations in hormone levels over time, identifying irregularities and predicting optimal fertility windows.
2. Basal Body Temperature (BBT):
– Markers: BBT charting helps track the slight temperature rise that occurs after ovulation.
– AI Application: AI can analyze BBT data to identify ovulation patterns and predict fertile periods based on temperature fluctuations.
3. Menstrual Cycle Length:
– Markers: Regularity or irregularity in menstrual cycles.
– AI Application: AI algorithms can process historical menstrual cycle data to predict future cycles, identifying potential fertility issues or predicting optimal conception times.
4. Cervical Mucus Changes:
– Markers: Changes in cervical mucus consistency and appearance during the menstrual cycle.
– AI Application: AI can analyze images or descriptions of cervical mucus to identify patterns and predict fertile or infertile phases.
5. Ovulation Prediction Kits (OPKs):
– Markers: LH surge detected by OPKs.
– AI Application: AI can interpret OPK results and predict ovulation timing based on historical data, helping users plan conception attempts.
6. Body Mass Index (BMI):
– Markers: Underweight or overweight conditions can affect fertility.
– AI Application: AI can analyze BMI data along with other physiological markers to assess overall fertility health and provide personalized recommendations.
7. Medical History and Conditions:
– Markers: Conditions such as polycystic ovary syndrome (PCOS) or endometriosis.
– AI Application: AI can analyze medical history data and identify correlations between specific conditions and fertility outcomes, aiding in personalized fertility predictions.
8. Genetic Markers:
– Markers: Genetic factors affecting fertility.
– AI Application: AI can analyze genetic data to identify potential risks or predispositions to fertility issues, offering personalized insights.
9. Lifestyle Factors:
– Markers: Factors like smoking, alcohol consumption, and stress.
– AI Application: AI can integrate lifestyle data to assess its impact on fertility, providing recommendations for lifestyle modifications.
10. Ultrasound Imaging:
– Markers: Visual assessment of reproductive organs and structures.
– AI Application: AI can analyze ultrasound images to identify abnormalities or conditions affecting fertility.
To implement AI in fertility prediction, a diverse dataset comprising these physiological markers should be used for training and validation. Machine learning models, such as neural networks, support vector machines, or decision trees, can then be applied to make predictions based on new data. It’s crucial to consider ethical and privacy considerations when working with sensitive health data. Additionally, collaboration with healthcare professionals is essential to ensure accurate interpretations and effective integration into clinical practice.
IV. Enhancing Embryo Selection through AI
A. Traditional Methods of Embryo Selection
1. Manual grading by embryologists
Manual grading by embryologists refers to the assessment and evaluation of embryos during in vitro fertilization (IVF) procedures. Embryologists are specialized professionals who work in reproductive medicine and are responsible for handling and cultivating embryos in a laboratory setting.
The manual grading process involves carefully examining embryos under a microscope and assigning them a grade based on various morphological criteria. The goal is to select the healthiest and most viable embryos for transfer to the uterus, increasing the chances of a successful pregnancy. The grading process typically includes assessing factors such as:
1. Cell Number and Division: Embryos are monitored for the number of cells they have and how evenly they are dividing. A certain number of cells at specific stages of development are considered optimal.
2. Cell Symmetry: The symmetry of cell division is crucial. Embryos with cells that are evenly distributed and uniform in size are generally considered healthier.
3. Fragmentation: Fragmentation refers to the presence of small, irregularly shaped fragments within the embryo. Increased fragmentation can be a sign of compromised embryo quality.
4. Blastocyst Development: In later stages of development, embryos form a blastocyst, a fluid-filled structure with an inner cell mass. The expansion and quality of the blastocyst are important factors in grading.
5. Degree of Expansion: The extent to which the blastocyst has expanded can provide information about its developmental stage and potential for implantation.
6. Trophectoderm Quality: The outer layer of cells in the blastocyst, known as the trophectoderm, plays a role in implantation. Its quality is assessed during grading.
Embryologists use a grading system that typically involves assigning a numerical or letter grade to each of these criteria. For example, a commonly used grading system for blastocysts is from 1 to 6, with 1 being the best quality and 6 being the poorest.
It’s important to note that while embryo grading provides valuable information, it is not a guarantee of pregnancy success. Other factors, such as the health of the uterus, the age and health of the woman, and overall fertility factors, also play a role in the success of IVF.
2. Limitations and subjectivity in embryo selection
Embryo selection, particularly in the context of assisted reproductive technologies like in vitro fertilization (IVF), involves choosing embryos with desirable traits or characteristics before implantation. While this process can be beneficial for preventing genetic disorders and increasing the chances of a successful pregnancy, it also comes with limitations and subjective elements. Here are some key points to consider:
1. Incomplete Genetic Information: Embryo selection relies on the analysis of genetic information, typically through preimplantation genetic testing (PGT). However, this testing may not provide a comprehensive picture of the embryo’s genetic makeup, and certain genetic conditions may go undetected.
2. Mosaicism: Mosaicism refers to the presence of different genetic information in different cells of the same embryo. This can make it challenging to accurately assess the genetic health of an embryo, as a biopsy from one part of the embryo may not represent the entire genetic composition.
3. Ethical Concerns: The subjective nature of selecting embryos with specific traits raises ethical concerns. Deciding which traits are considered desirable or undesirable can be influenced by cultural, societal, or personal beliefs, leading to potential discrimination or the creation of societal norms based on certain characteristics.
4. Complex Traits and Polygenic Disorders: Some traits and conditions are influenced by multiple genes and environmental factors, making it challenging to predict and select embryos based on these factors alone. Polygenic disorders, in particular, may not be easily identified through current genetic testing methods.
5. Limited Predictive Power: Genetic testing provides information about the presence or absence of specific genetic mutations, but it may not predict complex traits, intelligence, or other characteristics with high accuracy. Many traits are influenced by a combination of genetic and environmental factors.
6. Unintended Consequences: The pursuit of specific traits in embryo selection may lead to unintended consequences, such as the reduction of genetic diversity within the population or the reinforcement of certain stereotypes and biases.
7. Emotional Impact: The process of embryo selection may have emotional implications for prospective parents, as they may grapple with decisions about which embryos to implant or discard. The emotional aspect of choosing among potential future children raises ethical questions about the value placed on specific traits.
8. Legal and Regulatory Challenges: There are ongoing debates and variations in regulations surrounding embryo selection. Different countries and regions may have different legal frameworks and guidelines, adding complexity to the ethical considerations involved.
In conclusion, while embryo selection holds promise for preventing genetic disorders and improving the success rates of assisted reproductive technologies, it is essential to be aware of its limitations and the subjective nature of the process. Ethical considerations and ongoing advancements in technology and genetic understanding will continue to shape the landscape of embryo selection.
B. AI-driven Embryo Selection
1. Computer-aided image analysis
Computer-aided image analysis in embryo selection using AI is a cutting-edge application of technology in the field of assisted reproductive technology (ART). This approach leverages artificial intelligence (AI) algorithms to analyze and assess embryos during in vitro fertilization (IVF) procedures. The goal is to improve the chances of successful implantation and ultimately increase the success rates of IVF treatments.
Here’s how computer-aided image analysis in embryo selection using AI typically works:
1. Image Acquisition:
– High-quality images or videos of developing embryos are captured using specialized microscopy techniques.
– Time-lapse imaging is often used to monitor the progression of embryo development in real-time.
2. Data Preprocessing:
– Images are preprocessed to enhance quality, remove noise, and standardize features for consistent analysis.
– The preprocessing step is crucial for ensuring that the AI algorithms receive clean and standardized input data.
3. Feature Extraction:
– Relevant features, such as embryo morphology, cell number, symmetry, and other characteristics, are extracted from the images.
– AI models can learn to recognize patterns in these features that are indicative of embryo viability.
4. Training AI Models:
– AI models, often based on deep learning techniques, are trained using a dataset of labeled embryos.
– The labeled dataset includes information on the developmental outcomes of embryos, such as successful implantation or pregnancy.
5. Prediction and Decision Support:
– Once trained, the AI model can analyze new, unseen embryos and provide predictions regarding their viability.
– Clinicians use this information as additional data in the decision-making process for selecting embryos with the highest likelihood of success.
6. Clinical Integration:
– The AI system is integrated into the clinical workflow of fertility clinics.
– Clinicians review the AI-assisted predictions alongside other relevant patient data to make informed decisions about which embryos to transfer.
Benefits of using AI in embryo selection:
– Increased Accuracy: AI models can analyze large datasets and identify subtle patterns that may not be apparent to the human eye, leading to more accurate predictions.
– Consistency: AI systems provide consistent and objective assessments, reducing variability between different embryologists.
– Time Efficiency: Automated analysis speeds up the evaluation process, allowing clinicians to make decisions more efficiently.
– Improved Success Rates: By selecting embryos with higher potential for successful implantation, the overall success rates of IVF treatments may be improved.
It’s important to note that while AI has shown promise in this field, it should be used as a complementary tool to support clinical decision-making rather than replacing the expertise of fertility specialists. Ongoing research and clinical validation are essential to ensure the reliability and safety of AI-assisted embryo selection methods.
2. Machine learning algorithms for embryo grading
Embryo grading in the context of assisted reproductive technologies (ART) involves assessing the quality of embryos to select the most viable ones for implantation. Machine learning algorithms can be employed to automate and improve the accuracy of embryo grading. Here are some commonly used machine learning techniques for this purpose:
1. Convolutional Neural Networks (CNNs):
– Application: CNNs have been successful in image recognition tasks, making them suitable for analyzing images of embryos.
– Usage: Input embryo images can be processed through a CNN to extract features and make predictions about their quality.
2. Support Vector Machines (SVMs):
– Application: SVMs can be used for classification tasks, including embryo grading.
– Usage: Features extracted from embryo images or other relevant data can be used to train an SVM for accurate classification of embryos into different quality categories.
3. Random Forests:
– Application: Random Forests are an ensemble learning method that can be applied to classification tasks.
– Usage: They can be used to handle non-linear relationships and capture complex patterns in embryo grading data.
4. Neural Networks:
– Application: More general neural network architectures, beyond CNNs, can be employed for embryo grading.
– Usage: Designing neural networks with appropriate architectures and training them on labeled embryo data can enable accurate predictions.
5. Decision Trees:
– Application: Decision trees can be used for classification and regression tasks.
– Usage: Decision trees can be trained on features extracted from embryos to create a decision-making process for grading embryos.
6. K-Nearest Neighbors (KNN):
– Application: KNN is a simple, instance-based learning algorithm.
– Usage: It can be applied to embryo grading by considering the quality of nearby embryos in the feature space.
7. Ensemble Methods:
– Application: Techniques such as bagging and boosting can improve the robustness and performance of machine learning models.
– Usage: Combining multiple models, possibly of different types, can lead to more accurate embryo grading.
8. Recurrent Neural Networks (RNNs):
– Application: RNNs are useful for sequential data, such as time-series information related to embryo development.
– Usage: If available, time-dependent features can be incorporated into an RNN for improved embryo grading.
When applying machine learning algorithms for embryo grading, it’s crucial to have a well-labeled dataset with accurate and reliable annotations. Additionally, the interpretability of the model’s predictions is important, especially in medical applications, to ensure that clinicians can trust and understand the grading decisions made by the algorithm. Regular updates and refinements to the model based on new data and insights from the field are also recommended for ongoing improvement.
C. Success Stories and Clinical Evidence
1. Case studies demonstrating improved outcomes
There are several ongoing studies and emerging trends indicating the potential benefits of using artificial intelligence (AI) in in vitro fertilization (IVF). Keep in mind that the field of AI and IVF is rapidly evolving, and there may be more recent developments. Here are a few potential case studies that could highlight improved outcomes when using AI in IVF:
1. Ivy AI in Embryo Selection:
– Ivy is an AI system developed to analyze time-lapse images of developing embryos during IVF. By examining factors such as cell division patterns and other morphological features, Ivy aims to improve embryo selection for transfer.
– Case studies may demonstrate how clinics utilizing Ivy AI experience increased pregnancy rates and reduced time to pregnancy compared to traditional methods.
2. Predictive Analytics for Implantation Success:
– Some AI platforms leverage predictive analytics to assess the likelihood of embryo implantation success. These systems analyze various patient and treatment parameters to provide personalized predictions.
– Case studies might showcase how clinics using these predictive analytics tools achieve higher implantation rates and optimize treatment protocols based on individual patient profiles.
3. Closed-Loop Control Systems in Ovarian Stimulation:
– AI-based closed-loop control systems are designed to dynamically adjust the dosage of hormones during ovarian stimulation based on the patient’s response. These systems aim to enhance oocyte yield while minimizing the risk of ovarian hyperstimulation syndrome.
– Case studies could illustrate how clinics implementing AI-driven closed-loop control systems achieve better-controlled ovarian stimulation, leading to improved IVF success rates.
4. Genetic Screening and AI in Preimplantation Genetic Testing (PGT):
– AI algorithms are being employed to analyze genetic data from embryos during PGT. These algorithms can help identify chromosomal abnormalities and select embryos with the highest potential for a successful pregnancy.
– Case studies may highlight how the integration of AI into PGT workflows results in more accurate and efficient genetic screening, leading to improved pregnancy outcomes.
5. Personalized Treatment Plans with AI:
– AI can analyze vast datasets, including patient medical history, genetic information, and treatment outcomes, to identify patterns and predict the most effective treatment plans for individual patients.
– Case studies may demonstrate how personalized treatment plans generated by AI contribute to higher success rates and reduced treatment cycles for couples undergoing IVF.
It’s essential to stay updated with the latest research and developments in the field of AI and IVF, as new case studies and advancements continue to emerge. Additionally, consider checking recent publications, medical journals, and fertility clinics’ reports for the most current information.
2. Comparative analysis with traditional methods
In vitro fertilization (IVF) is a complex and widely used assisted reproductive technology that helps couples struggling with infertility to conceive. The integration of artificial intelligence (AI) in IVF has shown promise in optimizing various aspects of the process. Here’s a comparative analysis between traditional methods in IVF and those incorporating AI:
1. Embryo Selection:
– Traditional Methods: Embryo selection is typically based on morphological assessments by embryologists. This involves observing the visual characteristics of embryos under a microscope.
– AI-Assisted Methods: AI algorithms can analyze more extensive datasets, including time-lapse videos of embryo development, and provide quantitative assessments. Machine learning models can learn patterns associated with successful embryo development, potentially improving selection accuracy.
2. Time-Lapse Monitoring:
– Traditional Methods: Embryo development is observed at specific time points, limiting the amount of information available to embryologists.
– AI-Assisted Methods: Continuous time-lapse monitoring allows for more detailed analysis of embryo development. AI algorithms can identify subtle changes and patterns not easily discernible by human observation.
3. Predictive Analytics:
– Traditional Methods: Reliance on historical and observational data, with predictions based on experience and expertise.
– AI-Assisted Methods: Machine learning models can analyze large datasets to identify predictive factors for successful implantation. This allows for more personalized treatment plans based on individual patient characteristics.
4. Ovarian Stimulation:
– Traditional Methods: Fixed protocols for ovarian stimulation may not account for individual variations in patient response.
– AI-Assisted Methods: AI can analyze patient data to optimize ovarian stimulation protocols, taking into account individual factors such as age, hormone levels, and previous treatment outcomes.
5. Risk Assessment:
– Traditional Methods: Limited ability to assess the risk of complications during pregnancy.
– AI-Assisted Methods: AI can analyze patient data to identify potential risks, such as the likelihood of preterm birth or complications during pregnancy, allowing for more informed decision-making.
6. Patient Selection and Counseling:
– Traditional Methods: Decisions based on clinical judgment and experience.
– AI-Assisted Methods: AI can assist in patient selection by analyzing comprehensive patient data, helping to identify those most likely to benefit from IVF and providing personalized counseling.
7. Cost Optimization:
– Traditional Methods: Resource allocation and decision-making based on historical data and general protocols.
– AI-Assisted Methods: AI can optimize resource allocation, reducing unnecessary tests and procedures, thus potentially lowering the overall cost of IVF treatment.
It’s important to note that while AI offers several potential benefits in the field of IVF, ongoing research is crucial to validate the effectiveness and safety of AI-assisted approaches. Additionally, ethical considerations, data privacy, and the need for human expertise in decision-making remain important aspects to be addressed in the integration of AI in reproductive medicine.
V. Addressing Ethical and Legal Concerns
A. Privacy and Data Security
1. Handling sensitive patient information
Handling sensitive patient information in the context of in vitro fertilization (IVF) when using artificial intelligence (AI) requires careful consideration and adherence to strict privacy and security protocols. Here are some key aspects to consider:
1. Data Encryption:
– Ensure that all patient data, including medical records, personal information, and any other sensitive details, are encrypted both during transmission and storage. This helps protect the information from unauthorized access.
2. Secure Storage:
– Store patient data in secure databases or systems that comply with industry standards for data security. Implement access controls to restrict who can view, modify, or interact with the data.
3. Access Controls:
– Implement strict access controls to ensure that only authorized personnel have access to sensitive patient information. This includes using role-based access controls and regularly reviewing and updating access permissions.
4. Anonymization and De-identification:
– Before using AI algorithms, consider anonymizing or de-identifying patient data to reduce the risk of identifying individuals. This involves removing or encrypting personally identifiable information (PII) from the dataset.
5. Data Minimization:
– Only collect and use the data necessary for the intended purpose. Avoid unnecessary or excessive data collection to minimize the risk associated with handling sensitive information.
6. Consent and Transparency:
– Obtain informed consent from patients regarding the use of their data for AI applications in IVF. Clearly communicate the purposes, risks, and benefits of using AI in the fertility treatment process.
7. Audit Trails:
– Implement audit trails to track and monitor access to patient information. This helps in identifying any unauthorized access or potential security breaches.
8. Regular Security Audits:
– Conduct regular security audits to identify and address vulnerabilities in the system. This includes both technical assessments and procedural reviews to ensure compliance with security standards.
9. Compliance with Regulations:
– Be aware of and comply with data protection regulations and standards relevant to healthcare, such as the Health Insurance Portability and Accountability Act (HIPAA) in the United States or the General Data Protection Regulation (GDPR) in the European Union.
10. Vendor Security:
– If utilizing third-party AI solutions or services, ensure that vendors adhere to strict security and privacy standards. Conduct due diligence on their security practices and ensure contractual agreements include data protection clauses.
11. Employee Training:
– Train healthcare staff and AI system users on privacy and security best practices. Create awareness about the importance of safeguarding patient information and maintaining confidentiality.
Remember that the ethical and responsible use of AI in healthcare, especially in reproductive technologies like IVF, is crucial for building trust with patients and ensuring the highest standards of privacy and security. Regularly reassess and update security measures to stay aligned with evolving threats and regulatory changes.
2. Compliance with data protection regulations
Compliance with data protection regulations is crucial when implementing AI in any field, including In Vitro Fertilization (IVF). In many regions, data protection laws are designed to safeguard individuals’ privacy and ensure responsible and ethical use of their personal information. Here are some key considerations for ensuring compliance with data protection regulations in the context of AI in IVF:
1. Informed Consent:
– Obtain clear and informed consent from individuals before collecting and processing their personal data. Provide comprehensive information about how AI will be used in the IVF process, the types of data involved, and the potential implications.
2. Data Minimization:
– Collect only the necessary data for the intended purpose and avoid excessive data collection. Minimize the use of sensitive information unless explicitly required for the AI algorithms in IVF.
3. Purpose Limitation:
– Clearly define the purpose for collecting and using personal data in the context of AI in IVF. Ensure that the data is used only for the specified purpose and not for unrelated activities.
4. Data Security:
– Implement robust security measures to protect personal data from unauthorized access, disclosure, alteration, and destruction. Encryption, access controls, and secure storage are essential components of data security.
5. Data Transparency:
– Be transparent about how AI algorithms operate in the IVF process. Provide individuals with understandable information about how their data will be used to avoid any unintended surprises.
6. Data Retention and Deletion:
– Establish clear policies for data retention and deletion. Retain personal data only for as long as necessary and ensure that it is securely deleted when no longer needed.
7. AI Algorithm Explainability:
– Ensure that the AI algorithms used in IVF are explainable and understandable. Transparency in the decision-making process helps individuals comprehend how their data influences the outcomes.
8. Accountability:
– Assign responsibility for compliance with data protection regulations within the IVF process. Establish internal mechanisms to monitor and enforce adherence to privacy principles.
9. International Data Transfers:
– If data is transferred across borders, ensure compliance with international data transfer regulations. Implement safeguards such as Standard Contractual Clauses (SCCs) or other approved mechanisms.
10. Regular Audits and Assessments:
– Conduct regular privacy audits and assessments to evaluate the effectiveness of data protection measures. This includes reviewing AI algorithms, data processing activities, and security protocols.
11. Stay Informed:
– Keep abreast of developments in data protection regulations to ensure ongoing compliance. Laws may evolve, and it’s crucial to adapt practices accordingly.
It’s important to consult with legal experts and relevant authorities to ensure compliance with specific data protection laws in the region where the IVF process is conducted. Additionally, involving ethicists and patient advocates in the development and implementation of AI in IVF can contribute to a more responsible and patient-centric approach.
B. Informed Consent and Patient Autonomy
1. Ensuring patients are well-informed about AI integration
Ensuring that patients are well-informed about AI integration in In Vitro Fertilization (IVF) is crucial for establishing trust, managing expectations, and promoting transparency. Here are some key strategies to achieve this:
1. Educational Materials:
– Develop clear and concise educational materials explaining how AI is integrated into the IVF process.
– Use layman’s terms to describe the role of AI, its benefits, and how it enhances the efficiency and effectiveness of IVF procedures.
– Provide visuals and examples to make complex concepts more accessible.
2. Patient Workshops and Seminars:
– Organize workshops or seminars where patients can learn about AI in IVF from healthcare professionals.
– Allow for open discussions, questions, and answers to address individual concerns and misconceptions.
3. Personalized Consultations:
– Offer one-on-one consultations with healthcare providers to discuss AI integration in IVF on a personal level.
– Tailor information based on the patient’s specific situation, ensuring relevance and addressing individual concerns.
4. Online Resources:
– Create a dedicated section on your clinic’s website or patient portal that provides comprehensive information about AI in IVF.
– Include videos, testimonials, and frequently asked questions (FAQs) to enhance understanding.
5. Informed Consent Process:
– Integrate information about AI into the informed consent process for IVF treatments.
– Ensure that patients understand the implications, benefits, and potential risks associated with the use of AI technologies.
6. Collaboration with Patient Advocacy Groups:
– Collaborate with patient advocacy groups to disseminate information about AI in IVF.
– Leverage these groups to create a supportive community where patients can share experiences and insights.
7. Continuous Communication:
– Establish a continuous communication channel between healthcare providers and patients throughout the IVF journey.
– Provide updates on the use of AI, share success stories, and address any emerging concerns.
8. Transparent Policies:
– Be transparent about the clinic’s policies regarding the use of AI in IVF.
– Clearly communicate how patient data is handled, ensuring privacy and security.
9. Training for Healthcare Providers:
– Ensure that healthcare providers are well-trained to communicate effectively about AI with patients.
– Continuous education for healthcare professionals will enable them to address patient queries with confidence.
10. Feedback Mechanism:
– Establish a feedback mechanism where patients can share their experiences and express any concerns related to AI integration in IVF.
– Use feedback to improve communication strategies and address any gaps in information.
By employing these strategies, clinics can empower patients to make informed decisions about IVF treatments involving AI, fostering a positive and trusting relationship between healthcare providers and patients.
2. Balancing technological advancements with patient rights
Balancing technological advancements with patient rights in In Vitro Fertilization (IVF) when incorporating AI involves addressing ethical, legal, and social considerations to ensure that the use of technology aligns with the well-being and autonomy of individuals undergoing fertility treatments. Here are some key principles and considerations:
1. Informed Consent:
– Ensure that patients are fully informed about the use of AI technologies in their IVF treatment.
– Clearly communicate the potential benefits, risks, and limitations of AI applications.
– Obtain explicit and informed consent from patients before implementing AI in their fertility treatments.
2. Transparency and Explainability:
– AI algorithms used in IVF should be transparent and explainable, allowing patients to understand how decisions are made.
– Patients should have access to information about the algorithms, data inputs, and the reasoning behind treatment recommendations.
3. Data Privacy and Security:
– Implement robust data protection measures to safeguard sensitive patient information.
– Comply with data privacy regulations to ensure that patient data is handled securely and ethically.
4. Algorithmic Bias and Fairness:
– Regularly assess AI algorithms for biases that may impact the fairness of treatment recommendations.
– Mitigate any identified biases to ensure equitable access to fertility treatments for all patients.
5. Human Oversight and Intervention:
– Retain a significant level of human involvement in the decision-making process, allowing for medical professionals to override AI recommendations when necessary.
– Maintain the doctor-patient relationship and ensure that medical professionals remain central to the fertility treatment process.
6. Regulatory Compliance:
– Adhere to existing regulations and standards governing medical treatments, ensuring that the use of AI in IVF complies with established guidelines.
– Advocate for the development of specific regulations addressing AI applications in reproductive medicine.
7. Continual Monitoring and Evaluation:
– Regularly assess the performance and impact of AI technologies in IVF through ongoing monitoring and evaluation.
– Adjust practices based on feedback and emerging evidence to enhance patient outcomes and protect their rights.
8. Education and Awareness:
– Educate both healthcare professionals and patients about the capabilities and limitations of AI in IVF.
– Foster awareness of patient rights and empower individuals to make informed decisions about their fertility treatments.
9. Accessibility and Affordability:
– Strive to make AI-enhanced IVF treatments accessible to a diverse range of individuals, addressing concerns about equity and affordability.
10. Ethical Guidelines and Committees:
– Establish ethical guidelines for the use of AI in reproductive medicine and create committees that include medical professionals, ethicists, and patient representatives to oversee and guide decision-making.
By incorporating these principles, practitioners and policymakers can work towards achieving a balance between technological advancements and patient rights in IVF treatments involving AI. This approach aims to promote ethical practices, patient autonomy, and positive reproductive outcomes.
VI. Challenges and Future Directions
A. Technical Challenges
1. Integration with existing IVF technologies
Integrating Artificial Intelligence (AI) with existing In Vitro Fertilization (IVF) technologies presents both opportunities and challenges. AI has the potential to enhance the efficiency, accuracy, and success rates of IVF procedures, but there are several considerations and hurdles to overcome. Here are some challenges and future directions in integrating AI with IVF technologies:
Challenges:
1. Data Privacy and Security:
– AI systems in IVF rely on vast amounts of sensitive patient data. Ensuring the privacy and security of this data is crucial to comply with regulations and build trust among patients.
2. Ethical Considerations:
– Decisions made by AI systems may raise ethical concerns, such as the selection of embryos based on certain traits. It’s essential to establish ethical guidelines and frameworks to guide the use of AI in reproductive technologies.
3. Interpretability and Explainability:
– AI algorithms, especially deep learning models, are often considered black boxes. Understanding and explaining the decisions made by these systems are essential for gaining acceptance from both clinicians and patients.
4. Data Quality and Standardization:
– Inconsistent and incomplete data can hinder the performance of AI models. Establishing standardized protocols for data collection and ensuring data quality are essential for the reliability of AI applications in IVF.
5. Integration with Existing Workflows:
– Integrating AI seamlessly into existing IVF workflows can be challenging. Clinicians may resist changes to established practices, and the integration process should be user-friendly and not disrupt the daily routine.
6. Validation and Clinical Trials:
– Before widespread adoption, AI algorithms need rigorous validation through clinical trials to demonstrate their effectiveness, safety, and positive impact on IVF outcomes.
Future Directions:
1. Predictive Analytics and Personalized Medicine:
– AI can be used to develop predictive models for individual patient outcomes, allowing for personalized treatment plans based on specific characteristics and genetic factors.
2. Optimizing Treatment Protocols:
– AI can analyze large datasets to identify patterns and optimize treatment protocols. This includes predicting the best time for embryo transfer and adjusting medication dosages for better outcomes.
3. Robotics and Automation:
– Integrating AI with robotic systems can enhance the precision and efficiency of procedures, such as sperm injection and embryo transfer, leading to improved success rates.
4. Enhanced Imaging and Diagnostics:
– AI can improve the analysis of imaging data, such as embryo morphology, increasing the accuracy of embryo selection. This could lead to better implantation rates and reduced risk of multiple pregnancies.
5. Patient Engagement and Education:
– AI-powered tools can be developed to educate patients, providing them with personalized information about their fertility journey and helping them make informed decisions.
6. Long-term Follow-up and Outcome Monitoring:
– AI can assist in monitoring the long-term health outcomes of children born through IVF, providing valuable insights into the safety and effectiveness of different procedures.
7. International Collaboration and Standards:
– Establishing international standards and fostering collaboration among researchers, clinicians, and policymakers can promote the responsible and ethical use of AI in IVF on a global scale.
In summary, while there are challenges in integrating AI with existing IVF technologies, addressing these issues can pave the way for significant improvements in the field, leading to more personalized and effective fertility treatments. Continued research, collaboration, and adherence to ethical guidelines are crucial for the successful integration of AI in reproductive medicine.
2. Ensuring AI systems are adaptable to diverse patient populations
Ensuring that AI systems used in the context of in vitro fertilization (IVF) are adaptable to diverse patient populations is crucial for providing equitable and effective healthcare. Here are some considerations and strategies to achieve this:
1. Diverse Data Representation:
– Ensure that the AI system’s training data includes a diverse range of patient demographics, including age, ethnicity, and medical history.
– Pay attention to variations in fertility factors across different populations, such as genetic predispositions, lifestyle, and cultural practices.
2. Ethical Data Collection:
– Collect data ethically and with respect for patient privacy, ensuring that diverse populations are represented without bias.
– Obtain explicit consent from patients for the use of their data in training AI models.
3. Cultural Sensitivity:
– Develop AI systems that take into account cultural differences related to fertility treatment preferences, beliefs, and practices.
– Consider language preferences and ensure that the AI system can communicate information in multiple languages.
4. Robust Algorithms:
– Use algorithms that are robust and adaptable to different physiological variations among patient populations.
– Continuously update and refine algorithms based on ongoing research and feedback from diverse patient groups.
5. Clinician Collaboration:
– Involve clinicians from diverse backgrounds in the development and validation process to ensure the AI system aligns with real-world clinical scenarios.
– Encourage collaboration between AI researchers, clinicians, and fertility specialists to address unique challenges faced by different patient populations.
6. User Interface and Experience:
– Design user interfaces that are intuitive and culturally sensitive, ensuring that patients from diverse backgrounds can easily interact with the AI system.
– Provide educational resources in multiple languages to enhance patient understanding and engagement.
7. Regular Audits for Bias:
– Conduct regular audits to identify and rectify biases within the AI system that may affect different patient populations.
– Implement strategies to minimize biases in data collection and algorithmic decision-making.
8. Accessibility:
– Ensure that the AI system is accessible to individuals with varying levels of technological literacy, education, and socioeconomic status.
– Consider the availability of resources such as internet connectivity and mobile devices across different populations.
9. Adaptability to Medical Advances:
– Design AI systems that can adapt to evolving medical knowledge and technological advancements, ensuring that the latest evidence-based practices are incorporated.
10. Global Collaboration:
– Foster collaboration with international organizations, research institutions, and healthcare providers to gather diverse datasets and insights.
By incorporating these considerations into the development and implementation of AI systems for IVF, you can help ensure that these technologies are adaptable to the diverse needs of patient populations, promoting inclusivity and equitable healthcare outcomes.
B. Ethical Considerations
1. Mitigating biases in AI algorithms
Addressing biases in AI algorithms, especially in the context of In Vitro Fertilization (IVF), is crucial to ensure fair and equitable outcomes. Biases can arise from various sources, including biased training data, algorithmic design, or societal biases present in the data. Here are some strategies to mitigate biases in AI algorithms related to IVF:
1. Diverse and Representative Training Data:
– Ensure that the training data used to develop AI algorithms is diverse and representative of the population undergoing IVF. This includes diversity in terms of age, ethnicity, socio-economic status, and other relevant factors.
– Regularly update training data to reflect changes in the population and medical practices.
2. Transparency and Explainability:
– Make efforts to enhance the transparency and explainability of AI algorithms. Provide clear documentation on how the algorithms make decisions to help users understand the underlying processes.
– Encourage transparency in the data collection and labeling process to identify and rectify potential biases.
3. Bias Detection and Evaluation:
– Implement tools and techniques for detecting biases in both the training data and the AI algorithms themselves. Regularly evaluate the model’s performance across different demographic groups to identify and rectify any disparities.
– Use metrics that account for fairness and balance in predictions, such as demographic parity or equalized odds.
4. Ethical Review and Oversight:
– Establish an ethical review board or committee to oversee the development and deployment of AI algorithms in the context of IVF. This can help ensure that potential biases are identified and addressed before widespread use.
– Involve diverse stakeholders, including medical professionals, ethicists, and representatives from the communities affected by IVF, in the decision-making process.
5. Bias Mitigation Techniques:
– Explore and implement bias mitigation techniques such as re-sampling, re-weighting, or adversarial training to reduce biases in predictions.
– Consider using fairness-aware machine learning techniques that explicitly address fairness concerns during the model training process.
6. User Feedback and Iterative Improvement:
– Encourage feedback from users and stakeholders to identify any unintended biases or adverse effects of the AI algorithms in real-world settings.
– Implement a continuous improvement process, where the algorithms are iteratively updated based on feedback and new insights.
7. Legal and Regulatory Compliance:
– Ensure compliance with existing laws and regulations related to privacy, data protection, and non-discrimination in healthcare. Stay informed about emerging regulations that may impact AI applications in the field of IVF.
8. Collaboration and Industry Standards:
– Collaborate with other organizations, researchers, and industry experts to share best practices and collectively work towards mitigating biases in AI algorithms for IVF.
– Support the development of industry standards that promote fairness, transparency, and accountability in AI applications related to reproductive health.
By adopting a multi-faceted approach that combines technical, ethical, and regulatory measures, it’s possible to mitigate biases and promote the responsible use of AI algorithms in the field of IVF.
2. Ensuring transparency and accountability in AI decision-making
Ensuring transparency and accountability in AI decision-making in the context of In Vitro Fertilization (IVF) is crucial to uphold ethical standards, protect patient rights, and build trust in the use of AI technologies in reproductive medicine. Here are some key considerations:
1. Explainability and Interpretability:
– AI algorithms should be designed to provide clear and understandable explanations for their decisions. This is especially important in medical contexts like IVF, where patients and healthcare professionals need to comprehend the reasoning behind AI recommendations.
– Make efforts to ensure that the AI models used in IVF are interpretable, allowing clinicians to understand the factors influencing the decision-making process.
2. Ethical Guidelines and Standards:
– Establish and adhere to ethical guidelines and standards specific to AI applications in IVF. Collaborate with medical professionals, ethicists, and relevant stakeholders to develop and update these guidelines regularly.
– Ensure that AI algorithms align with established ethical principles, including patient autonomy, beneficence, non-maleficence, and justice.
3. Informed Consent:
– Clearly communicate to patients the use of AI in their IVF treatment and obtain informed consent. Patients should be aware of how AI is utilized, the potential implications, and their right to opt-out if they are uncomfortable with AI involvement in their care.
4. Data Privacy and Security:
– Implement robust data privacy and security measures to protect sensitive patient information. Comply with relevant data protection regulations, such as GDPR or HIPAA, to safeguard the confidentiality of patient data used in AI decision-making.
5. Human Oversight:
– Maintain a balance between AI-driven decision-making and human expertise. Human clinicians should have the ability to override AI recommendations, especially in situations where ethical or clinical judgment is essential.
6. Bias Mitigation:
– Regularly assess and address biases within AI algorithms to prevent discriminatory outcomes. Ensure that training data used to develop AI models is representative and diverse to avoid perpetuating existing biases.
7. Continuous Monitoring and Auditing:
– Establish a system for continuous monitoring and auditing of AI algorithms in IVF. Regularly assess the performance, accuracy, and fairness of the models to identify and rectify any issues promptly.
8. Collaboration and Stakeholder Engagement:
– Involve a multidisciplinary team of experts, including clinicians, ethicists, data scientists, and patient advocates, in the development and deployment of AI systems in IVF. Encourage open dialogue and collaboration to ensure a well-rounded perspective.
9. Education and Training:
– Provide education and training to healthcare professionals involved in IVF about the use of AI. This includes understanding the capabilities and limitations of AI, as well as its ethical implications.
10. Transparency Reports:
– Publish transparency reports detailing the AI algorithms’ functionality, data sources, and performance metrics. This enhances accountability and allows the public and medical community to assess the reliability and fairness of the technology.
By incorporating these principles, the use of AI in IVF can be transparent, accountable, and aligned with ethical considerations, ultimately benefiting both patients and healthcare providers. Regularly reassessing and updating these practices will be essential to keep pace with evolving ethical standards and technological advancements.
C. Future Prospects
The integration of artificial intelligence (AI) with reproductive technologies has the potential to revolutionize various aspects of reproductive healthcare and family planning. Here are some potential areas of integration:
1. Fertility Prediction and Optimization:
– AI can analyze vast amounts of data, including genetic information, lifestyle factors, and health records, to predict fertility windows more accurately.
– Machine learning algorithms can help optimize fertility treatments by predicting the success rates of different procedures based on individual patient data.
2. Assisted Reproductive Technologies (ART):
– AI can assist in the selection of viable embryos during in vitro fertilization (IVF) by analyzing morphological features, genetic markers, and other relevant data.
– Predictive analytics can help optimize the timing of embryo transfer to increase the chances of successful implantation.
3. Personalized Medicine for Reproductive Health:
– AI can analyze genetic data to identify potential risks for reproductive health issues and provide personalized recommendations for preventive measures.
– Tailored treatment plans can be generated based on individual genetic profiles, optimizing outcomes for fertility treatments.
4. Telemedicine and Remote Monitoring:
– AI-powered applications can facilitate remote monitoring of reproductive health, allowing users to track menstrual cycles, ovulation, and other relevant parameters.
– Telemedicine platforms can use AI to provide personalized advice and support for fertility-related concerns.
5. Genetic Screening and Counseling:
– AI can enhance the efficiency and accuracy of genetic screening for hereditary diseases, helping couples make informed decisions about family planning.
– Virtual genetic counselors powered by AI can provide guidance and support based on individual genetic profiles.
6. Predictive Analytics for Pregnancy Complications:
– AI algorithms can analyze health data to predict the likelihood of pregnancy complications, allowing for early intervention and personalized care plans.
– Continuous monitoring of health parameters during pregnancy using wearable devices can provide real-time data for AI analysis.
7. Ethical Considerations and Counseling:
– AI can assist healthcare providers in addressing ethical considerations associated with reproductive technologies, ensuring that patients are well-informed about potential risks and benefits.
– AI-powered virtual counselors can offer emotional and psychological support to individuals and couples undergoing fertility treatments.
8. Research and Development:
– AI can accelerate research in reproductive medicine by analyzing vast datasets, identifying patterns, and generating hypotheses for further investigation.
– Drug discovery for reproductive health issues can benefit from AI-driven approaches, potentially leading to the development of more effective treatments.
However, it’s essential to approach the integration of AI with reproductive technologies with careful consideration of ethical, privacy, and regulatory concerns. Striking a balance between technological advancements and ethical considerations is crucial to ensure the responsible and beneficial application of AI in reproductive healthcare.
VII. Conclusion
A. Summary of Key Findings
1. AI’s impact on IVF success rates
Artificial intelligence (AI) has the potential to positively impact in vitro fertilization (IVF) success rates by enhancing various aspects of the fertility treatment process. Here are some ways in which AI can contribute to improving IVF outcomes:
1. Embryo Selection:
– AI algorithms can analyze images of embryos and identify characteristics associated with viability, assisting embryologists in selecting the most promising embryos for transfer.
– Machine learning models can learn from historical data to predict which embryos are more likely to result in successful pregnancies, improving the overall success rates of IVF.
2. Optimizing Treatment Protocols:
– AI can analyze large datasets of patient information, including demographic details, medical histories, and treatment outcomes, to identify patterns and optimize IVF treatment protocols.
– Personalized treatment plans based on individual characteristics and responses to previous cycles can lead to more effective and tailored fertility interventions.
3. Predicting Ovulation and Fertility Windows:
– AI can analyze various factors, such as menstrual cycle data, hormonal levels, and other relevant parameters, to predict ovulation and fertility windows more accurately.
– By improving the timing of procedures, AI can increase the chances of successful fertilization and implantation during IVF.
4. Monitoring and Feedback:
– Continuous monitoring of patients during IVF cycles, using AI-driven systems and wearable devices, can provide real-time feedback on physiological parameters.
– This allows for prompt adjustments to treatment plans based on individual responses, potentially enhancing the likelihood of successful outcomes.
5. Genetic Screening and Risk Assessment:
– AI can assist in the analysis of genetic data to identify potential risks and abnormalities in embryos, improving the selection of embryos with a higher chance of successful implantation and healthy pregnancies.
– Predictive analytics can help assess the risk of genetic disorders and guide decision-making regarding the use of pre-implantation genetic testing (PGT).
6. Patient Counseling and Support:
– AI-powered virtual assistants can provide ongoing support and guidance to patients undergoing IVF, offering information, answering questions, and addressing concerns throughout the treatment process.
– Emotional and psychological support can contribute to better patient experiences and potentially impact IVF success rates.
It’s important to note that while AI shows promise in improving IVF outcomes, its implementation should be done cautiously, considering ethical considerations, patient privacy, and the need for human oversight. Additionally, the success of AI applications in reproductive medicine relies on the availability of high-quality data for training and validation, as well as collaboration between AI experts, fertility specialists, and other healthcare professionals. Ongoing research and clinical validation are essential to ensure the reliability and safety of AI-driven interventions in the field of assisted reproduction.
B. Implications for the Future
The use of Artificial Intelligence (AI) in In Vitro Fertilization (IVF) has the potential to bring about significant advancements and improvements in reproductive medicine. Here are some potential implications for the future:
1. Enhanced Predictive Analytics:
– AI algorithms can analyze large datasets, including patient health records, genetic information, and historical IVF outcomes, to identify patterns and predict the likelihood of success for a particular patient undergoing IVF.
– Improved predictive analytics can help clinicians tailor treatment plans, optimizing the chances of a successful pregnancy and reducing the number of unsuccessful cycles.
2. Personalized Treatment Plans:
– AI can assist in developing personalized treatment protocols based on individual patient characteristics, medical history, and genetic information.
– Tailoring treatment plans to the specific needs of each patient can improve the overall success rates of IVF and minimize the physical and emotional toll of multiple unsuccessful attempts.
3. Time and Cost Efficiency:
– AI can streamline the IVF process by automating routine tasks, such as data analysis, embryo selection, and monitoring.
– Increased efficiency can lead to reduced costs for both healthcare providers and patients, making fertility treatments more accessible to a broader population.
4. Embryo Selection and Genetic Screening:
– AI-powered image analysis can aid in the selection of the most viable embryos by assessing morphological features.
– Genetic screening using AI can help identify embryos with genetic abnormalities, improving the chances of a successful pregnancy and reducing the risk of miscarriage.
5. Patient Counseling and Support:
– AI can provide personalized counseling and support to couples undergoing IVF, offering information about the process, managing expectations, and providing emotional support.
– Virtual assistants or chatbots powered by AI can be available 24/7 to address patient queries and concerns.
6. Research and Innovation:
– AI can accelerate research in reproductive medicine by analyzing vast amounts of genetic and clinical data, leading to the discovery of new insights and treatment options.
– The integration of AI can foster innovation, driving the development of novel techniques and technologies in assisted reproductive technologies.
7. Ethical Considerations:
– The increased use of AI in IVF raises ethical considerations, such as the responsible and transparent use of patient data, potential biases in algorithms, and the need for clear guidelines on AI-assisted decision-making in reproductive medicine.
8. Regulatory Challenges:
– The integration of AI into IVF may present regulatory challenges, including the need for standardized protocols, guidelines, and oversight to ensure the safety and efficacy of AI applications in fertility treatments.
As AI continues to advance, its integration into IVF holds great promise for improving outcomes, personalizing treatments, and making fertility care more accessible. However, it also requires careful consideration of ethical, legal, and regulatory implications to ensure responsible and equitable implementation.
The integration of Artificial Intelligence into In Vitro Fertilization represents a groundbreaking advancement in the field of reproductive medicine. As AI technologies continue to evolve, the role they play in enhancing fertility assessment and embryo selection holds immense potential for improving success rates and providing new hope for couples facing infertility challenges. However, careful consideration of ethical and legal implications is crucial to ensure that these technological advancements are implemented responsibly and with a focus on patient well-being and autonomy.